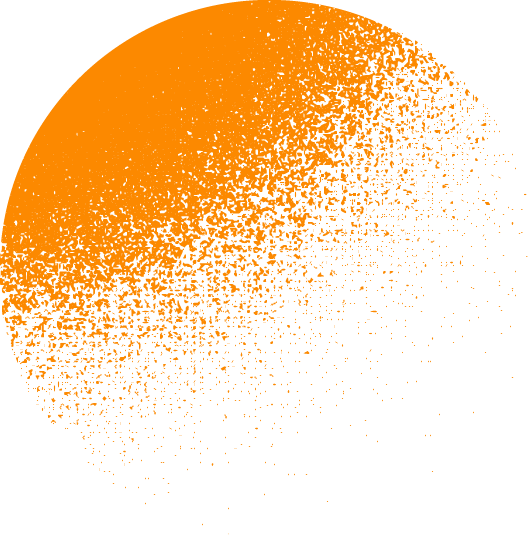
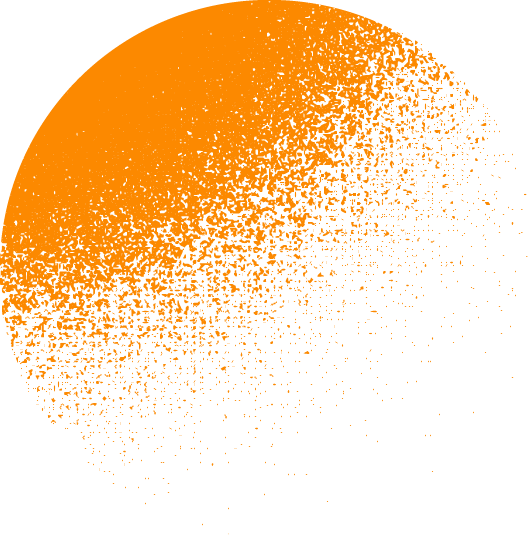
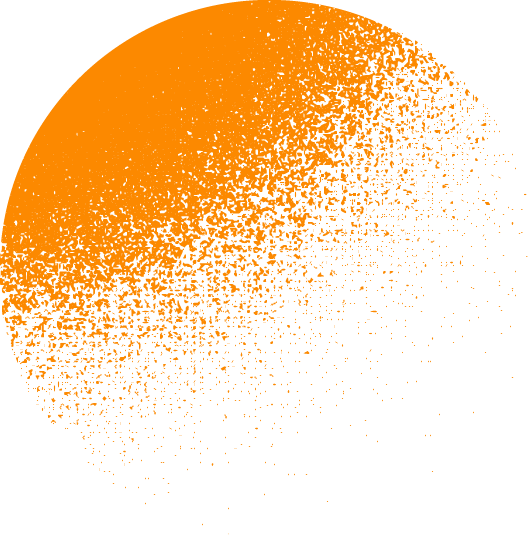
Probability and Statistics for Deep Learning
CSE-41305
Deep Learning is often called “Statistical Learning” and approached by many experts as statistical theory of the problem to find the best model approximation from a given collection of data. These models are produced today by Generative Deep Learning, which is a key to unlocking the latest sophisticated form of AI, comparable to human’s form of intelligence. AI is already present in our everyday lives, for example face recognition at airports, background generation in video conferencing, etc. You cannot understand CHAT GPT and Large LLMs if you don’t understand generative modeling and deep learning.
This course will introduce important concepts of probability theory and statistics which are the foundation of today’s Deep Learning. It will cover many important algorithms and modelling used in discriminative and generative deep learning models. In addition, the course will introduce tools and underlying mathematical concepts of data interpretation that work with specific models of deep neural networks. Upon completion of this course, you will have acquired the background in probability and statistics necessary for Machine Learning, and have the ability to use TensorFlow to create and train neural networks for specific practical problems.
Course Highlights:
- Knowledge of probability theory basic rules: conditional probability, joint probabilities, marginal probability
- Quantify events: Random Variables and becoming familiar with representing big data
- Important Probabilistic Distributions for Deep Learning
- Important Statistical Learning concepts: Entropy, KL divergence
- Statistical Estimators: MLE and MAP
- Logistic Regression and probabilistic models for classification (Kernels)
- Bayesian concept learning
- Discriminative models:
- Supervised Learning Algorithms: Decision Trees and Random Forest
- Unsupervised Learning Algorithms: K-Means & Gaussian models (mixture model)
- Structured Probabilistic Models: Probabilistic Graphical Model, Markov Model
- Generative modeling: what are the properties of good generative model, (transformers, LLMs, Multimodal)
- Utilize TensorFlow 2.9 or PyTorch for real world example
Software: TensorFlow 2.9 Probability, PyTorch, Python 3.9.
We will use tools which are opensource and you will run it on UCSD Extended Studies' server using virtual environment setup for you with Jupyter notebook.
Course typically offered: Online in Winter, Spring and Fall quarters
Prerequisites: Basic knowledge of Linear Algebra - concept of vectors and matrices
Next steps: After completing of this course, consider taking other courses in the Machine Learning Methods, or Technical Aspects of Artificial Intelligence certificate program
More information: For more information about this course, please contact unex-techdata@ucsd.edu
Course Information
Course sessions
Section ID:
Class type:
This course is entirely web-based and to be completed asynchronously between the published course start and end dates. Synchronous attendance is NOT required.
You will have access to your online course on the published start date OR 1 business day after your enrollment is confirmed if you enroll on or after the published start date.
Textbooks:
All course materials are included unless otherwise stated.
Policies:
- No refunds after: 6/30/2025