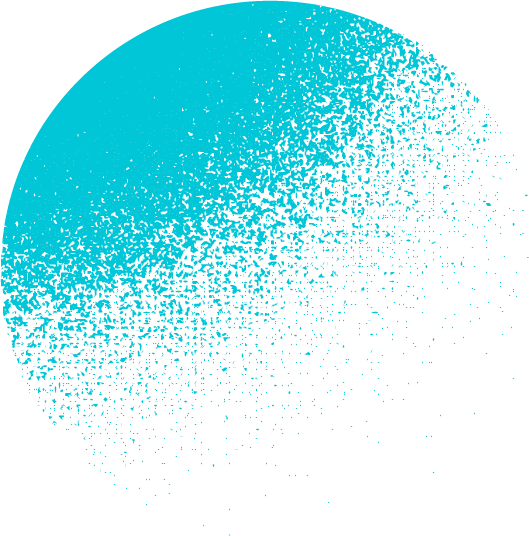
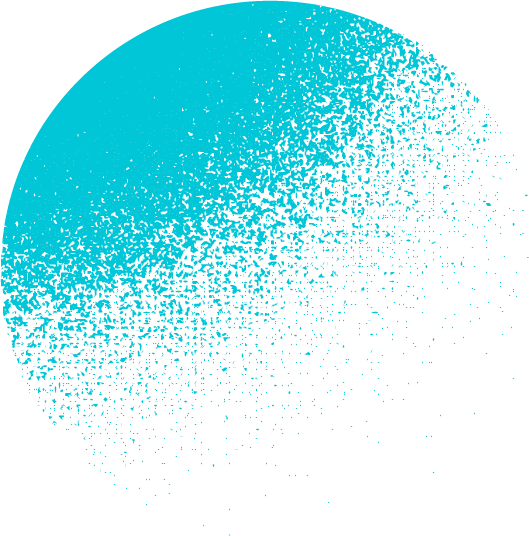
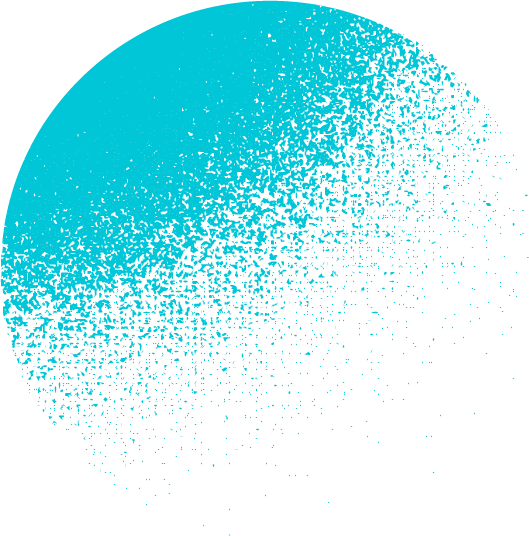
22 May 2025
What is Quantum Machine Learning?
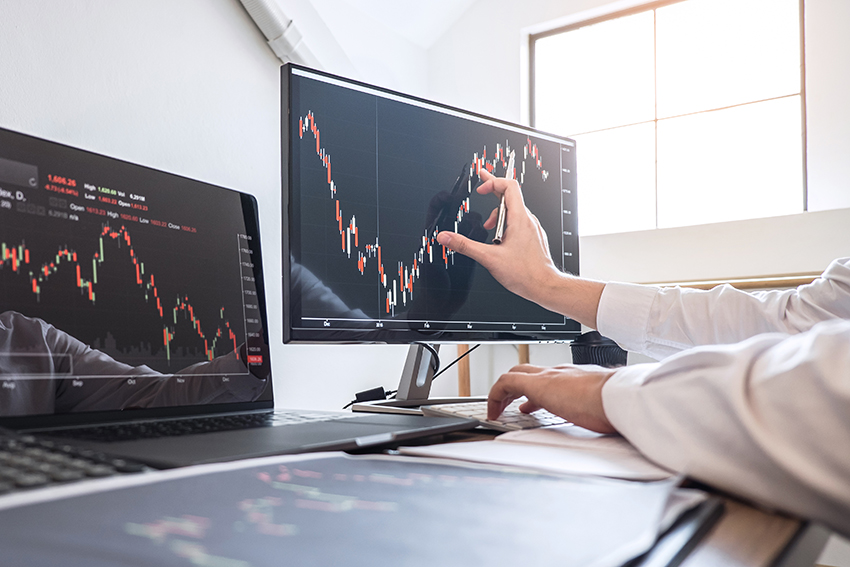
Quantum computing is no longer just a theory—it's beginning to transform machine learning and impact the world around us.
By merging quantum tech with AI, quantum machine learning (QML) is opening doors in areas like security and healthcare. With the field expanding fast, learning the essentials is a smart move. And, you don't need a PhD in physics or math to get up to speed.
Students and professionals can prepare for real-world quantum computing roles by building practical skills and a strong conceptual foundation.
Quantum computing might sound like science fiction, but it's quickly becoming reality—and it's already making waves in the world of machine learning.
From improving fraud detection to speeding up drug discovery, Quantum Machine Learning (QML) is enabling new breakthroughs across industries by combining the power of quantum computers with the intelligence of AI.
As the field rapidly evolves, its impact on various industries and daily life is becoming increasingly significant. For students and professionals ready to dive in, beginning with the fundamentals is a great place to start.
A Short History of Quantum Computing
Quantum computing is built on the strange and powerful rules of quantum physics—things like superposition (where particles can be in more than one state at once) and entanglement (where particles remain connected across distances).
Although the concept of quantum computing has existed since the 1980s, technology has only recently caught up. Thanks to advances in hardware and cloud-based platforms like IBM Q and Pennylane, quantum computing is no longer just a research topic—it's entering classrooms, labs, and commercial applications around the world.
Why Quantum Machine Learning?
Machine learning (ML) has already revolutionized how we work, live, and communicate. From personalized medicine to financial forecasting, classical machine learning algorithms help us make sense of vast amounts of data.
But quantum machine learning (QML) takes things a step further.
Quantum computers can process information in a fundamentally different way. Instead of working with bits (0s and 1s), they use qubits (pronounced, “q-bits”), which is short for quantum bits. Qubits are special because they can be 0, 1, or both at the same time. This allows quantum computers to handle and analyze complex data in a way that classical computers can’t—making them potentially much faster and more powerful for certain tasks.
In fact, some calculations that would take a classical computer thousands of years could be done in minutes with the right quantum algorithms.
What Can You Do with QML?
As the field grows, so do the career opportunities. Quantum computing is already being used in a range of fields to impact outputs and outcomes.
Some examples include:
- Healthcare – speeding up drug discovery and genetic research
- Finance – improving fraud detection and portfolio optimization
- Logistics – optimizing complex supply chains in real time
- Climate science – modeling weather systems and environmental changes
- Cybersecurity – developing next-generation encryption and data protection
Real-World Impacts of Quantum Machine Learning
Major companies are investing heavily in QML, signaling a strong demand for talent. For instance:
- IBM is pioneering QML research through its IBM Quantum platform and partnerships with universities and startups.
- Google has made headlines with quantum supremacy experiments and continues to explore QML applications in optimization and AI.
- Xanadu, a Toronto-based startup, is developing the Pennylane platform to make QML more accessible.
- Amazon Braket provides cloud-based access to quantum computing tools, including frameworks for QML.
These developments show that QML isn't just theoretical. It's shaping the next generation of technology jobs, products, and services.
Getting Started with QML—No PhD Required
For students and professionals curious about this fast-growing field, the course Intuitive Learning of Quantum Machine Learning at UC San Diego Extended Studies offers an accessible starting point. Rather than diving into heavy physics or math, it focuses on building intuition and practical skills.
The course is divided into four main parts:
- A clear overview of classical vs. quantum machine learning
- Why quantum speedups matter and how they work
- The foundations of major QML algorithms
- Hands-on practice using IBM Qiskit and Pennylane
Plus, the curriculum emphasizes hands-on practice. Using free tools like Qiskit and Pennylane, students can try out quantum algorithms on real quantum computers—no previous quantum experience required.
Looking Ahead: The Future of Quantum Computing
The potential of quantum computing is massive. As quantum processors become smaller and more powerful—possibly even reaching room-temperature functionality—the technology could soon be part of everyday smart devices.
With that shift comes a growing need for a quantum-literate workforce. But not everyone needs to be a quantum physicist. The Intuitive Learning of Quantum Machine Learning course is designed to prepare students for real-world quantum computing roles by building practical skills and a strong conceptual foundation.
In short: If you're curious about the future of computing and want to stay ahead of the curve, UC San Diego Extended Studies offers a solid entry point to your journey.
To learn more about the Intuitive Learning of Quantum Machine Learning course, contact unex-techdata@ucsd.edu.