By Kelly Davis
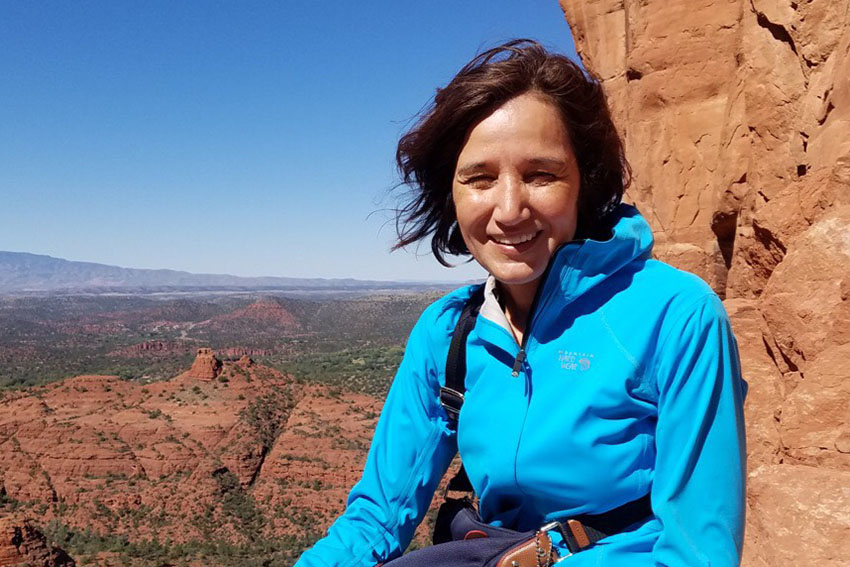
Name: Bilyana Aleksic
Course: Linear Algebra for Machine Learning
A computer with a mind of its own — like 2001’s HAL, for instance — was a story hook for countless 20th century sci-fi films. But in this century, it’s one step closer to reality through advances in artificial intelligence and “machine learning,” a type of programming that allows computers to learn from data to recognize patterns and make predictions. It’s already present in our daily lives; it’s how Netflix is able to point users to new films based on their past picks, how researchers sort through vast amounts of genetic data to come up with cancer therapies and how self-driving cars are able to react to road conditions as if a driver were behind the wheel.
Machine learning has existed for decades. In 1952, Arthur Samuel, who coined the term “machine learning,” taught an IBM computer to play checkers. The more the computer played the game, the better it became. Machine learning, then, is a lot like human learning — the more information, or data, we have on a subject, the easier it is to make informed choices. Machine learning just involves a whole lot more data.
Taught by Bilyana Aleksik, Extension's course in Linear Algebra for Machine Learning aims to help students learn the skills needed to understand and create machine learning algorithms. A former Qualcomm engineer with an interest in artificial intelligence, Aleksic says she was drawn to machine learning's practical — and groundbreaking — applications, like a microscope that can scan human tissue for cancer cells faster than a pathologist.
“You have a system that is already trained and knows to recognize a cell,” Aleksic explains.
Linear algebra is the most efficient way to represent this data. Though it’s been around for hundreds of years, it’s only been in the last decade that linear algebra has been applied to machine learning data mining.
“Machine learning engineers really need a good foundation in linear algebra,” Aleksic says. “Many of us didn’t have any idea when we were studying math at the university that we would use it so much in our everyday work. And now, really, it has become the groundwork, the base, to understand algorithms and to develop a model to extract information from huge amounts of data.”
Svetislav Maric, Extension’s director of engineering and IT, says the goal is for students to use the course as a springboard to more specialized machine learning classes, such as those offered in our Data Science courses.
Aleksic says it’s an exciting time to be working in this field. She hopes the course will lead to deeper dives into machine learning’s potential. Back when Arthur Samuel was teaching his IBM computer to play checkers, he was limited by the computer’s memory and processing speed. But now, that’s no longer an issue.
“This is the beginning, so we will see what is most useful, what area we’ll develop further: Is it image processing, is it the sound processing, or robotics? All this is happening right now, so we’ll learn as we go,” she says.
Was this article helpful? Let us know in the comments.
Updated October 9, 2019.